Data quality frameworks (DQF): A guide for stakeholders in pharma, healthcare and life sciences
Regulatory compliance is crucial in the pharmaceutical, healthcare and wider life sciences industries, where patient safety and outcomes underpin every decision and action. This means that data integrity and quality are crucial for effective patient care and scientific advancement.
A data quality framework (DQF) is an essential tool in achieving these goals. Anyone working in these industries should understand what a data quality framework is, how it operates and why it is important. Recent developments, such as the specific Data Quality Framework for EU medicines regulation, have made it crucial to keep up to date with changes that may affect compliance and quality.
What is a data quality framework?
A data quality framework is a structured set of standards, processes and guidelines designed to ensure data’s accuracy, consistency, completeness and reliability throughout its lifecycle. In the industries we are covering here, a DQF is vital for maintaining the integrity of clinical trial data, manufacturing records, adverse event reports and other critical information.
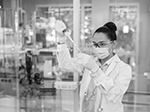
How can you gather, develop and maintain high-quality data?
Download our free guide for data quality stakeholders across pharma, healthcare and the life sciences now.
Download now
Key components of a DQF:
- Data integrity: Safeguarding the accuracy and consistency of data from creation to archiving.
- Data completeness: Ensuring enough data is gathered, measured and available for analysis and decision-making.
- Data consistency: Maintaining uniformity across data sets and formats.
- Data timeliness: Keeping data up-to-date and accessible when needed.
- Data accessibility: Ensuring data can be easily retrieved and used by relevant employees in different locations and even time zones.
Why are data quality frameworks needed?
The pharmaceutical, healthcare and life sciences industries are some of the most heavily regulated in the world – and with good reason. The stakes couldn’t be higher with patient care, safety and outcomes on the line. With this in mind, high-quality data is crucial for several reasons:
- Regulatory compliance:Countries worldwide are governed by their own regulatory bodies, such as the European Medicines Agency (EMA) and the U.S. Food and Drug Administration (FDA). These bodies have stringent rules and data quality standards for drug approval and monitoring patient outcomes with real-world data.
- Patient safety:Accurate and reliable data ensures that medicines are safe and effective for public use. Drug development and trials are rigorously administered, with data being an integral aspect of the process.
- Scientific integrity:High-quality data supports robust scientific research and innovation.
- Operational efficiency:Streamlined data processes reduce errors, save time and enhance decision-making, allowing excellence in quality and compliance.
How do data quality frameworks work?
A data quality framework operates through a series of steps and best practices designed to embed data quality into every stage of data handling:
- Policy development: Establishing clear policies and guidelines for data management.
- Training and education: Ensuring all stakeholders understand and adhere to data quality standards.
- Data collection and management: Implementing tools and systems for accurate and efficient data capture and storage.
- Monitoring and auditing: Regularly reviewing data processes to identify and correct issues.
- Continuous improvement: Using feedback and audit results to refine and enhance data quality practices.
Data Quality Framework for EU medicines regulation – a key change
The Data Quality Framework for EU medicines was released on December 12, 2023 by the European Medicines Agency (EMA) and the EU Heads of Medicines Agencies (HMA). This comprehensive document outlines essential definitions, principles and guidelines for ensuring the quality of data used in regulatory decision-making.
Why is the Data Quality Framework for EU medicines regulation needed?
The rapid development of technology and digitization of healthcare have created a revolution in the capture and curation of real-world data. Innovations including AI have created both opportunities and challenges to regulatory decision-making.
It was clear there was a need for methodology and guidelines which examined the suitability of new types of data for analysis and decision-making.
The HMA-EMA Joint Big Data Task Force and the Big Data Steering Group highlighted the necessity of a cohesive framework for data quality. They emphasized that such a framework is crucial for harnessing the potential of big data and facilitating sound regulatory decisions.
Scope and objectives:
- Consistency in DQ processes:Establish uniform data quality processes across the EU.
- Development of horizontal systems:Promote systems capable of handling diverse data sources.
- Support for automation:Introduce mechanisms for automated, data-driven regulatory decisions.
The framework provides definitions for data quality dimensions, general considerations for regulatory decision-making and a maturity model to guide the evolution of automated, data-driven regulatory decisions.
Ideagen’s role in data quality management
At Ideagen, we offer quality management solutions that help you implement robust data quality frameworks. Our software solutions facilitate the streamlining of workflows and processes, as well as the monitoring and tracking of data and stakeholder engagement through the creation of a quality culture. Crucially, Ideagen solutions are used by leading organizations across healthcare, pharma and life sciences to uphold patient safety and pursue continuous improvement.
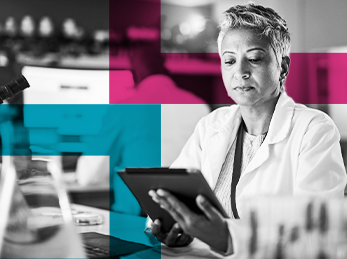
Why is data quality so important for pharmaceuticals?
Download our free guide today where we cover exactly why it is so essential in the production of safe, effective medicines.
Download now