What is the Data Quality Framework for EU medicines regulation?
The EU Data Quality Framework (DQF) for medicine regulation establishes criteria for a consistent and standardized approach to data quality in medicine regulation. It outlines essential considerations for data quality that are pertinent to regulatory decision-making, provides clear definitions for various dimensions of data quality and presents associated metrics.
The Data Quality Framework for EU medicines was released on December 12, 2023 by the European Medicines Agency (EMA) and the EU Heads of Medicines Agencies (HMA). This comprehensive document outlines essential definitions, principles and guidelines for ensuring the quality of data used in regulatory decision-making.
Want more information on the Data Quality Framework for EU medicines?
Download our free whitepaper that deep-dives into the regulation and what it means for EU medicines and, crucially, what it means in practice for your organization.
Download nowWhy did we need the Data Quality Framework?
The EU Data Quality Framework (DQF) for medicine regulation was established to address the increasing need for reliable and high-quality data in the regulatory process. As regulatory decisions significantly impact public health and safety, the formulation of standardized criteria ensures consistency in data evaluation, enhances transparency and bolsters confidence in outcomes.
By defining specific dimensions of data quality and their associated metrics, the DQF aims to facilitate better decision-making, ultimately leading to more effective regulatory practices and improved medicine safety standards.
Moreover, healthcare has become digitalized and technology is evolving at breakneck speed, bringing about a revolution in the capture and curation of real-world data (RWD). This is data relating to patient health status, patient outcomes and the delivery of healthcare.
These innovations, including artificial intelligence (AI), present new opportunities. But these opportunities add complexity to regulatory decision-making. New types of data are becoming available all the time, and it became very apparent that there was a need for methodology and guidelines to explore the suitability of this data for decision-making.
The HMA-EMA Joint Big Data Task Force and the Big Data Steering Group highlighted the necessity of a cohesive framework for data quality. They emphasized that such a framework is crucial for harnessing the potential of big data and facilitating sound regulatory decisions, leading to the development of the Data Quality Framework (DQF).
Purpose of the Data Quality Framework
The framework provides a structured set of terminology, definitions, principles and guidelines applicable to various data sources. Its primary goal is to characterize, assess and assure data quality (DQ) for regulatory decision-making.
Key objectives:
- Create consistency in data quality processes: The framework supports a uniform approach to data quality processes across the EU. This ensures that outcomes of regulatory assessments are reliable.
- Development of horizontal systems: The DQF promotes the creation of systems that can handle diverse data sources and types effectively.
- Automation for data-driven decisions: Introduce technology to support automated, data-driven regulatory decisions, enhancing efficiency and accuracy.
The Data Quality Framework takes existing definitions and recommendations from other data quality frameworks and extends them with the addition of the classification of quality dimensions and guidance on how to apply them with assessment criteria and guidelines.
The framework covers a wide range of regulatory activities and corresponding data types, including laboratory and preclinical data, bioanalytics and chemical and manufacturing control data.
Key components of the Data Quality Framework
- Data reliability: Ensuring that data remains accurate and unaltered throughout its lifecycle with robust documentation and security measures.
- Data extensiveness: This relates to the amount of data collected and ensures that the data gathered is sufficient.
- Data coherence: Harmonizing data from various sources to maintain uniformity. It relates to the consistency between different parts of a dataset.
- Data timeliness: Keeping data up-to-date and submitting it within required timeframes for prompt regulatory reviews.
- Data relevance: Assessing how a data set presents elements that can be used to answer a specific research question.
Best practices for implementing the Data Quality Framework
When implementing the Data Quality Framework, you should adopt the following best practices to ensure the process is effective:
- Establish clear policies and procedures: Develop comprehensive policies that outline data quality standards, roles, responsibilities and compliance requirements.
- Train staff and stakeholders: Investing in regular training and development ensures that employees at all levels understand their role in data quality protocols and maintaining compliance.
- Investment in technology: Investing in cutting-edge technology that streamlines and supports your quality management systems is essential.
- Conduct regular audits: Routine audits help maintain high standards and continuous compliance.
- Engage stakeholders: Foster collaboration among all stakeholders, including regulatory authorities and healthcare providers, to promote transparency and trust.
Ideagen's role in enhancing data quality
At Ideagen, we recognize the critical importance of data quality in the pharmaceutical sector. Our quality solutions are tailored to help you implement robust data quality frameworks and ensure compliance at all times.
We can help you navigate the complexity of the Data Quality Framework for European medicines regulation and provide solutions to streamline implementation. This includes tools to help create a robust, secure document system, tools for effective audits and effective training management – in addition to an array of additional features that help you establish unbreakable standards of quality in lie with the Data Quality Framework.
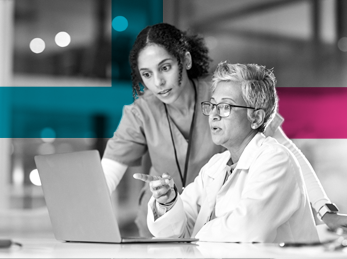
Looking for more advice on how to gather, develop and maintain high-quality data? Download our free guide for data quality stakeholders across healthcare and the life sciences now.
Download nowTags: